GSA challenge found industry machine-learning models can make do with limited training data
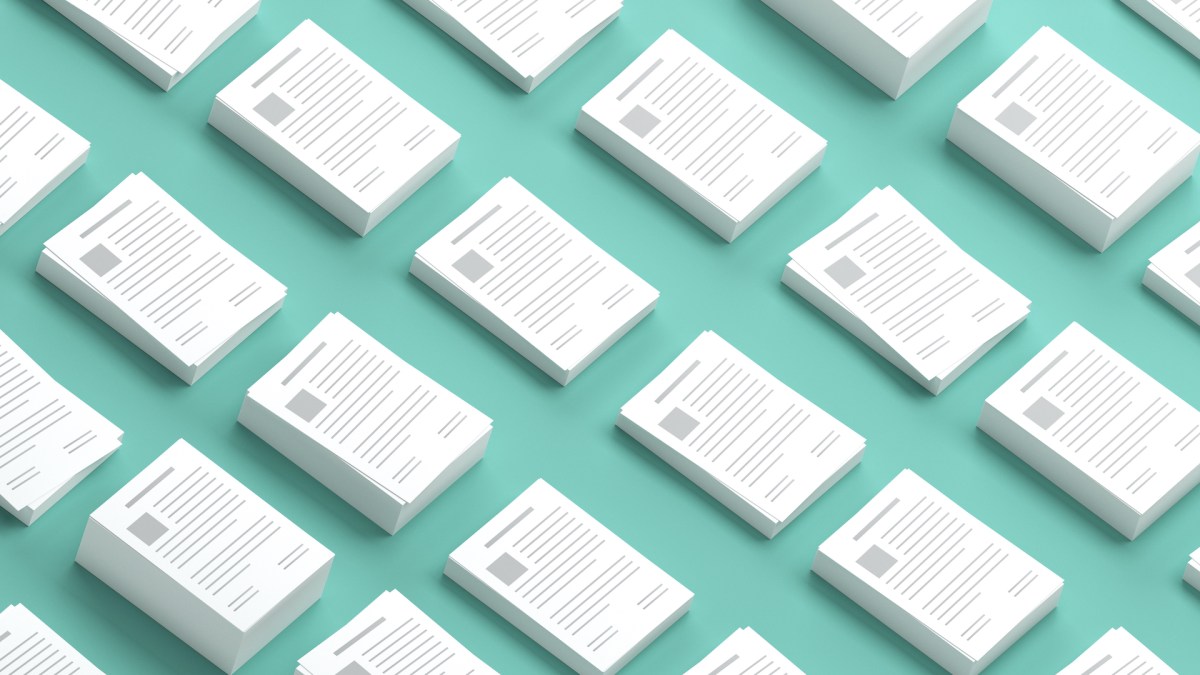
Several companies recently impressed the General Services Administration with their ability to use limited training data in supervised machine-learning (ML) models, says Ryan Day, director of the agency’s Digital Services Division.
As part of a recent contest on Challenge.gov, GSA tasked entrants with using ML or artificial intelligence to speed up reviews of software end-user license agreements (EULAs) — but the agency only provided several thousand rows of text with the use case.
“The first thing we learned was that industry could actually do this,” Day said Tuesday during the first day of FedTalks presented by FedScoop. “Our use case, going in we didn’t have any assumptions about whether or not it could be done with machine learning, but we found that it was a good fit.”
Normally supervised ML requires large amounts of data, but many of the 20 entries GSA received were “high quality” and used workaround techniques like transfer learning,
Transfer learning is used in natural language processing when open-source models are pre-trained with vast amounts of other text and then fine-tuned with data specific to an individual use case — in this case the EULAs.
Contracting officers (COs) generally take one to two weeks reviewing EULAs to ensure their terms and conditions align with federal law as part of the software acquisition process. COs may coordinate a legal review with the Office of General Counsel to negotiate the removal of problematic language.
The AI and Machine Learning Challenge allowed GSA to test current commercial practices, with multiple teams using the Bidirectional Encoder Representations from Transformers (BERT) language model for transfer learning.
Other teams found creative ways to augment and generate new training data, with one using a cloud tool to translate clauses into hundreds of other languages and then back into English, Day said. The new clauses had the same meaning but different diction and syntax, serving as new training data.
Yet another team proposed an application programming interface-based approach to breaking down Microsoft Word and PDF documents into clauses that predictions could be run on for determining viability.
Dev Technology placed first in October winning $15,000, while second-place Gaussian Solutions won $2,500 and third-place Team SoKat $2,500.
Meanwhile, GSA’s challenge allowed it to test commercial capabilities before developing proofs of concept, pilots and scaling into production.
“We can move some of the things that we learned into actual requirements from a business perspective, as well as a technology perspective, said Keith Nakasone, deputy assistant commissioner for acquisition in GSA’s Office of IT Category, at FedTalks. “So I think this is a good way to start; the challenge gave us some really good insight into the tools available.”
Ethical AI
As the Department of Defense, intelligence community and Department of Homeland Security begin exploring ML and AI technologies they’ve opted to establish ethical AI principles for their agencies to follow.
GSA is taking a slightly different approach by gathering ethical AI concepts from agencies participating in its AI Community of Practice, Nakasone said.
“It brings the agencies together so we can learn best practices, we can share information and also glean what we can do from creating templates and playbooks,” he said.
Industry has a role to play in informing GSA’s understanding of ethical AI as well, Nakasone said.
“Companies that are putting ethical principles out there for us to leverage is also another thing that we can consider from a contract and acquisition perspective,” he said.